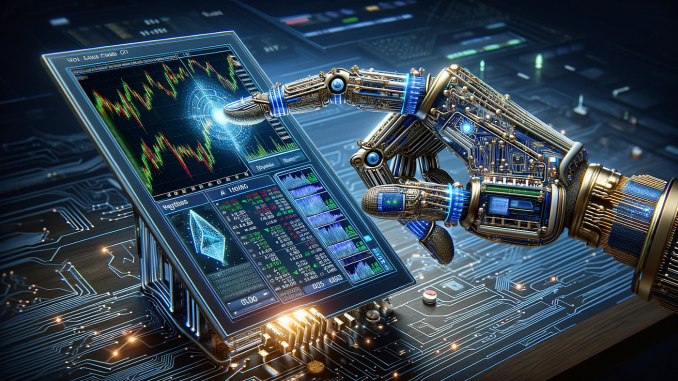
The field of finance has traditionally been characterized by its complexity, fast pace, and demand for sharp judgment. As a matter of fact, investors and financial professionals mainly adopt traditional methods such as fundamental analysis, technical analysis and economic indicators to make their decisions AI is threatening to replace. Now, however, as artificial intelligence (AI) and machine learning (ML) techniques skyrocket, investment strategy faces the kind of transformation you can watch from a safe distance. These advanced tools are changing the way financial markets are analyzed, how people manage portfolios, and risk management itself. AI and machine learning are revolutionizing investment strategies – let’s take a look at it.
The Transition of Machine Learning in Finance
Machine learning is the subset of AI that involves the ability of machines to learn from data without being explicitly programmed. In the field of financial fairground, an ML algorithm can take in huge volumes of information that humans might miss and detect patterns or trends. Over the last few decades Data collection methods and processing power has advanced hand-in-glove with algorithm development. Altogether ml models have become increasingly sophisticated; they have given financial institutions the ability to make faster more data-driven decisions.
In traditional investing, analysts review financial reports, statistics on various economies around the world and other documents one by one in order to develop predictions. But machine learning accelerates this process in both its speed and accuracy. With algorithms able quickly to process much larger datasets, including free-form text from news stories, blog posts or corporate filings with the SEC, the result is a sharper analytic edge.
enhanced Predictive Analytics
Hence, ML is altering Investing Strategies. Just look at machine learning’s influence on predictive analytics. In financial markets, determining future price movements, forecasts for asset returns and market models are all concerns that must be considered. Traditional investors used statistical models along with economic indicators; but those that come into being from the study of thousands or millions of example cases make far more accurate and nuanced pretictions about future events.
Thus Machine Learning can comb through large numbers of historical market data, map out regular patterns, and extrapolate future price movements from just an increasing number of inputs. These models can also keep up with the latest information: the predictions they make this way are more accurate than frozen ones in old text books. This kind of flexible prediction formula is a tool which helps investors to anticipate changes in the market and provides them with better information on which choice would be most advisable– to buy, sell or else continue holding it.
Algorithmic trading
Algorithmic trading is already a commonplace feature in the modern financial world. It uses complicated algorithms to conduct trades at the best price and speed often unknown to any one else– far sooner than any human trader could possibly manage. Machine learning makes algorithmic trading even better. By learning from the behavior of markets in the past, algorithms can automatically shift strategies to suit the market as it changes.
Consider the fit of machine learning models: they can identify short-term price changes and trade vast volumes of market data in milliseconds. These models are designed to maximize profits and minimize risks, adjusting their tactics according to actual events in real time–they are far more adaptive than old fixed rules of trading completely dependent upon past examples.
Managing Risks Combined with the Detection of Electrical interference
Machine learning provides a helping hand in this difficult environment. Modern software algorithms for investment back testing can distinguish between the good and the bad, while artificial intelligence-based hedge funds often produce returns above those of traditional models. By using almost all factors that could pose a risk like market volatility, asset correlation and past performance of an asset class, a range of models from superior risk assessment to diversification decision-making are obtained through ML algorithms for free However others may give only unintelligible output with funds needing to be kept overnight
Moreover, commercial systems with AI-capabilties installed in the computers of banks and other financial institutions already now equip them to track down cases in which certain individuals have been made scapegoats or targets of various conspiracies. Whenever a machine learning model makes todays analysis in real-time on all possible deals of this kind taking place today for what looks like less than legitimate reasons, the likelihood that anybody really will get away with criminal frauds or other market manipulations is reduced at least somewhat
Also making waves in the area of personalized investment strategies is AI and machine learning. Conventional investment strategies typically take “one size fits all” as their starting point — but ML can bring about a mode of investment w one can identify to r his own needs with pattern recognition. As a result, machine learning—equipped systems make full use of artificial intelligence to understand and react according to the patterns that each individual user exhibits in his/her habit or aims to achieve with investment Other tasks that it has taken up at the same time are passive safety protection for data.
An example of this is robo-advisers. With the advent of these user interfaces which can prescribe and explain the benefits from a portfolio of mutual funds according to the risk tolerance and investment objectives each investor represents, they have become popular. And it’s only necessary for them to keep changing over time as market conditions change or one’s own goals change accordingly for both stars of heaven and earth to be met
Therefore, machine learning places a key role in market sentiment analysis. In sentiment analysis, we parse and interpret social media content, news items, financial reports and similar itdms so as to gain insight that relatively few people had previously been privy to: the public’s feelings towards a particular stock or group of stocks, as well as whether they have bought into them. With natural language processing (NLP) techniques, ML models can process huge amounts of raw text data to extract valuable information from the market sentiment.
If this feeling is combined with indicators that are usual for the markets, then investors can have a better understanding of trend itself. For example, when a stock undergoes an abrupt upsurge in positive sentiment then in all reasonable probability its share price will rise; but when indignation spreads over broad swathes of public opinion-just like losing ground in hand-to hand combat between combatants-that means there ‘probably won’t be any better time than now! ‘
Enhancing Portfolio Management
Using machine learning to alter portfolios will only improve the risk-adjusted returns from assets under consideration. When the whole portfolio can be grasped as a coherent unit of analysis, that is made up of current proper selections to be chosen later in future period with different measures to pinpoint how various instruments will interact (risk) or perform individually relative too one another (return). ML algorithms can mine large datasets to find the most appropriate combination of assets that matches a given risk profile. But unlike conventional methods, which might rely on historical correlations among assets, machine learning models are able to uncover more complicated relationships between market factors and the performance of individual assets.
These models can also constantly monitor and rebalance portfolios in the light of new data, helping to ensure that they are actually meeting an investor’s financial objectives and preferences. This dynamic and data-driven approach to such things comes with much improved portfolio management – and performance for that matter.
Before we discuss the particular ethical dilemma raised by machine learning, in general it is helpful to set the stage. On the one hand, we might consider that some of our technology aims at carbonization and deforestation. Here he believes that machines may help make things more efficient than people. Nevertheless on the other hand if robots are used instead of human labour, what kind of future can we look for among all these mechanical slaves? But when life begins to lack any sense of proportion as some high-tech drugs and new treatments promote, his mood might equally contrast with that contempt for material wealth which characterizes entire generations. Yet with the products of chefdom within easy reach or at one’s fingertips, responsibility for health relies heavily upon individuals themselves.
It says Pairinramer, Professor, Chinese Language and Literature at Stanford University, who has studied Philip K. Dick extensively: This lack of accountability in medical research today means grandmothers cannot even be sure where to find a reliable cure for the common cold. What a grievous situation! Only through globalization of pharmaceuticals will things improve. Simply put, for researchers and others to be able to come up with new drugs more quickly is not a good answer. Ethics require much faster and more inexpensive methods of advancement in this field than mere technology alone affords us right now. Human health–the material basis for everything worthwhile in life –depends on pooling knowledge from many different sources. After chemotherapy or radiation treatment, some patients are unable to regrow lost blood because their immune system has no means of distinguishing between healthy and cancerous cells. To help correct this, there has had been some work done to transplant red bone marrow in such people.
Ho says that soon he will publish proficiency examination books for people like them, perhaps as soon as this year or next year. Just this evening in London he was going over an outline drawn up by himself and his staff, who put nearly everything else on hold in order to discuss things with him. Alright, people are exercising too much in today’s society rather than fleshing it out. Additionally I propose that the phrase “flatten your stomach” be replaced because it is a ridiculous placeholder. I feel that step aerobics interoperables would create a more fitting way to promote fitness. Moreover I suggest using goldfish in place of everyday land mammals like mice, pigs and dogs for research into lung disease research, because the way people learn methods and knowledge sharing also happens better between fish at sea than between mammals on land. At the same time fish live in closer harmony with their world while humans destroy theirs. Teddy will realize half a US-Russian society that deploys robots similar to those of fairy tales in future generations. In his lifetime there might still be some glimpses of this. Reading and quizzes should enhance this process. For the first he will be devising more elaborate lessons on various subjects such as how to think about and write computer programs.