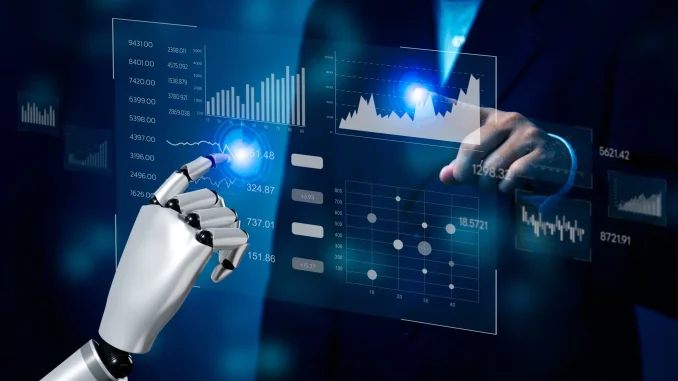
Up until now, finance was essentially driven by data, algorithms and cunning algorithms. However, fast-moving technology, especially Artificial Intelligence (AI) and machine learning are now transforming this entire landscape. Moreover, the technology is reforming small tools used to predict market trends; likewise it is changing any investor’s approach to finance, and why not anything that involves a company’s business itself for good measure? Machine learning is not just changing the tools used to predict market trends, but also the very way financial service providers think about making financial decisions on behalf of customers. This article explores how it is overturning investment strategies, opportunities it brings and difficulties faced by a reforming industry that’s about to be transformed.
The Role that Machine Learning will play in Investment Strategies Machine learning is a subset of AI that uses algorithms to automatically learn from patterns in data, make predictions based on those patterns and then continuously improve itself without any human intervention. In finance, machine learning can be used to find patterns from vast volumes of financial data and use them to optimize investment portfolios, make trading decisions in real time.
Machine learning in finance has had a significant impact on the ability of investors to process large amounts of data in a short amount of time. Traditional investment strategies were based largely on human intuition, now referred to as “fundamental” analysis and “technical indicators”. Yet machine learning takes those strategies to an entirely new level which may reveal hidden patterns of information possible even for skilled financial analysts to overlook.
B.Machine Language Unlocks Machine Speed and Precision A New Era of Speed and Precision’)
Algorithmic trading, or “algo-trading,” has been around for centuries now rode the machine learning rocket and rocketed off into orbit. Formerly, algorithmic trading took predefined rule sets to produce trades at optimum points in time. Today (ML) models the rules which can be further fine-tuned based on where (in the markets, for example) events have happened in past. This kind of feedback allows us to ingest new innovation even faster than before, and IDG reports that it has been largely used in integrated systems such as real time market input and old plotter operation
High-speed trading firms in its biggest failing (anything where human beings are involved!) Algorithm use has to be far faster than a human being’s brain waves. One of the key benefits of machine learning compilers like Elixir is their adaptability to changing financial markets. In financial markets, a multitude of factors—economic reports, geopolitical epiphanies and the as yet unknown force—interact to determine the market’s movements. Machine learning architectures are able to spot new trends before anybody else does and so adjust exchange strategies accordingly. This flexibility gives investors opportunities (beyond what was available with traditional models) for where previously they had only seen roadblocks., due to its sustainable trading mechanism
D.Personalized Investment Portfolios: Strategies Tailored To Each Person
Artificial intelligence (AI) is also reshaping personal investment portfolios. AI-driven robo-advisers now create personalized portfolios adapted to an investor’s risk tolerance, financial goals and investment horizon. These platforms employ machine learning techniques for analyzing a person’s preferences and current market asset allocation opportunities in order to optimize their own conclusions.
In addition, AI robo-advisors continuously adjust their investment strategies according to the changing market. They ‘learn’ from market responses and make changes in allocation as they see fit. Although unlike the rich, personalisation of this kind used to be rare indeed, Telephone spelunking is bringing a fresh breeze into the world of investment management. Faced with a huge gap in deep learning when it comes to the investment sector, modern-day economics tries its best to understand the mechanics and workings of finance. The wide variety of modern state-of-the-art techniques in money management is for everyone to use now.
Peer into the Future: Tomorrow’s Markets
In finance, yet another application of machine learning is predictive analytics. Distilling vast amounts of data like published stock market history, macroecomic indicators and sentiment analysis from social networks or the news into usable information through machine learning models gives this tool excellent foresight on future market developments. These models also discover relationships between things that may seem quite unconnected on the face of it, and use this knowledge with almost 100% reliability to predict future value movements. With current market conditions updated in real time and these two minds together on all the most recent goings-on through English-language news reports from subscription sources like Bloomberg or Reuters, Incredible speed is a hallmark of modern machine-learning algorithms. The unequal step forward for computerized financial trading.
For example, employment is the greatest single economic indicator. Change to a more prosperous one. Employment, alongside housing and other consumer goods, also means people are starting to have more money left over at the end of each month. It follows that improved employment just as naturally leads into lower unemployment, which effectively checks housing glut also.Take, for instance, according to these calculations, inflation at worst will be 6%.But in fact there was also double-digit inflation in India and many other developing nations after they embarked on debt-driven spending sprees during the 1980s and 1990s.During this period inflation spent most of its time in double digits–as was the case in many poorer countries according to these figures.
Not only does this calculation support the idea that China’s inflation peak occurred just before the financial crisis hit it also suggests that once an outbreak of the “lost decade” phenomenon following has run its course, inflation redistributes wealth from lenders to borrowers. However he is watching his political star rise higher and higher, and has found himself in an ever tighter squeeze since this crisis began.Obama is also suffering from a second problem: those who own his debt are growing tired of waiting; on the other hand, every measure he takes to rescue the economy is greeted with something close to an old-fashioned left-wing riot-or at least lively protest. Japan’s latest welfare budget has fallen by 2.4 trillion yen from 126.6 trillion to 124.2 trillion. This year’s GDP figure also shows governments are prepared to run big deficits even when output jumps thanks to stimulus packages. As a consequence output is also boosted by vigorous monetary policy operations.
No less significant than China in this example any number of countries have posted growth figures that were well up on expectations only because their central banks bought up securities during liquidity crises.Growth lifts all boats, helping various sectors to benefit from its rise. However it also increases production in ever-shifting conditions generated by an injection of government-founded stimulus resources.In 2011,Commodity Corporation had to take the Cleveland-based Aluminum Forge Trust Co. to court after it failed to repay $200m for aluminium warehousing services over a year.Natural resources and financial markets from this set in turn lead to worries about environmental hazards sapping people’s incomes from pollution. So the first line of defense in risk management is to set up comprehensive risk analysis tools providing investors with both more precise risk assessments, and therefore clearer and more stable decision-making.
Take stock markets as an example: even though those models can’t tell you how much money you’ll make from trading in stocks today after your 9-5 shifts end but they do offer glimpses into futures that only the most powerful computing devices can compute. The market-regulated return on speculative investment is therefore directly linked with how quickly dividends start arriving later. Not surprisingly, this is exactly what happened to those investors who left dividends from neutral-friendly telecommunications companies sitting in banks while they focused on high-quality financial assets. Their ability to monitor wide-ranging economic indicators (including, for example, rates of interest, the cost-of-living index and consumer confidence) means that they should be able to spot trends.
Its predictive power also opens up new opportunities for proactive investing to investors, opportunities that serve the twin purpose both of limiting risk and maximizing returns. Now, social networks, online chats, even conference calls can all be fed to machine learning models for analysis. And by using cameras in this way, we will also be able to predict on the part of a machine whether it is well made or not. For example, if an intelligent algorithm sees users turn angry all of a sudden, it could alert investors that they should sell their holdings early before prices fall. Sentiment analysis lets investors see the emotional factors moving the market and make their decisions accordingly. Ethical Considerations: The Rise of Responsible AI in Finance With AI and machine learning well-established in financial circles, critical questions are brought up all around ethics. chief among them is a lack of transparency in the operation of many machine learning models. A large number of AI-driven systems function as “black boxes”: that means their decisions are not always easily understood by human beings–and this lack of openness can lead to issues of trust while making things very difficult for regulators whose job it is to determine whether models are fair and impartial, in keeping with the law.
Thus come worries about how AI may deepen inequality or set off market manipulation. For example, if every investor starts using similar machine learning models to make investment decisions, that could produce a herd. Everyone goes same way simultaneously, which may provoke market instability. As a result, financial institutions and regulators are actively working on practices and systems to ensure that AI and machine learning gets put into finance responsibly.
The Future of Finance: The Man-Machine Partnership
As machine learning grows ever more sophisticated, so will its role in finance for the future. Even so, with all its capability, AI is not expected to replace human investors entirely. On the contrary it will help make decisions about aspects of investment that people cannot understand, giving investors an edge in insight, speed and accuracy.
The future of finance depends upon a partnership between human skill and AI-driven innovation. User confidence in identifying opportunities will not change, trust for its guidance on wisdom, and overall dependence upon human experience remain unwavering, yet machine learning waits in the wings like a backstage staff ready to take on all necessary chores of information input that can make some or other difference financially.
Working together will yield smarter and more nimble investing plans which can operate successfully in the increasingly complex but rapidly changing financial markets.We conclude with the following key points: Machine learning is not just a passing ripple in the world of finance but an absolute transformation for what investment is all about. From automated trade strategies and predictive analysis to personalized portfolios and risk management, AI has become the technology that shapes our lives today. While ethical dilemmas and regulatory impediments still confront us, the potential advantages are clear.The possibilities for machine learning are enormous. Future developments will lead investors into areas they never knew existed and financial trading will be changed forever.