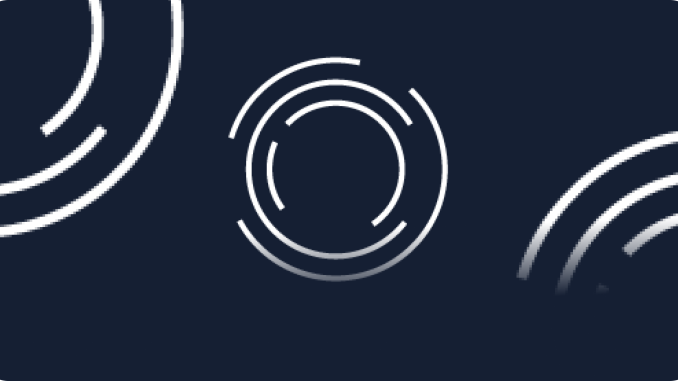
In the world of underwriting especially in insurance, finance and banking has typically been a menagerie of people with knowledge and experience. Finally, the human expert and the assessment of risk made manually. Just a single underwriter might use a number of indices such as health information and financial backgrounds in order to decide how risky it is (to what degree the company or indeed any given individual will be) Let the new power of artificial intelligence (short for AI) and machine learning (ML) overturn this procedure.
This change not only increases efficiency but also improves accuracy in a huge way. Artificial intelligence in underwriting and risk assessment is now speeding up not only the process but also introducing more sophisticated risk modelling. For insurance companies, this is undoubtedly a very nearly perfect bargain. Traditinal process of underwriting we may, however, perhaps better first understand the traditional process before exploring how AI is causing underwriting to evolve. In insurance underwriting, companies protect against risks and assess whether to issue a policy is right. Also up to what extent they are willing and able to accept such policies. This is where the underwriters will read: some bizanthe data such as so many Medical Records that a person is alive and healthy and therefore able to get insured, Credit Scores and Financial Reports devises without which they are unable product enough income for mortgages or auto loans.
Property assessments to show whether houses or property are worth consideration This is achieved manually and, though proven reliable, still comes with its share of problems. It can be time-consuming, vulnerable to human error or bias, and requires much manpower and investment. Furthermore, the Information Age is seeing a deluge of data that human underwriters simply cannot manage efficiently by themselves (see Chapter 4). There is is no other way but for complexity to come. How Machine Learning Changes the World of Risk Prediction Machine learning falls within the sphere of Artificial Intelligence, where computers themselves learn from patterns and arrive at decisions without human intervention. In insurance underwriting, ML algorithms are now being taught using massive volumes of past data so that they can predict risk at greater speed and accuracy.
Better Data Processing and Analysis By using ML systems, it is possible to process enormous quantities of data much faster than humanly feasible. This includes structured financial data and unstructured data such as clinical notes or social media activity which would ordinarily be very difficult to categorize. By analysing data types of this nature, ML can capture subtle trends that a human underwriter may not see, leading to fuller risk apprehension.
Risk Scoring and Predictive Analysis In traditional insurance underwriting, risk scores are determined by past history and expert opinion. With machine learning, this business of scoring risk is enormously variable. The more new data they process, AI systems predict better what will happen next than humans do. For instance, in health insurance underwriting AI can use genetic data or patterns of life-style to better determine rates for chronic disease than the standard actuarial tables has a right to inspire.
Blaze Underwriting Systems, which is an AI-based underwriting technology, is capable of handling for the underwrite more than 80% of the routine operations and processes involved in risk assessment. This is because many tasks that would otherwise end up in the hands of humans providing support for human judgment –such as dada entry and preliminary risk calculations are already being taken over by machines. Underwriters therefore have time to deal with the rare cases that require a personal touch.
Automation Through Computerization For insurers, this means quicker policy approvals, lower operational costs and delighted customers.
The Elimination Of Human Bias One of the major benefits of using AI in the field of underwriting is that it can help to reduce subjective factors. Even the best human underwriters may be influenced by conscious or unconscious prejudices arising from their own backgrounds and status. Machine learning models, properly trained, make decisions solely based on data patterns. So this kind analysis can help eliminate many typos and terms of reference change, for fairer endings.
Real-time Data Updates Now AI systemsupported underwriting can re-evaluate risk wherever necessary during a policy term. Traditional underwriting lacks this agility, for example, in terms of real time data. Take life insurance:Collectivities of lifeforms on earth have always tried to get around the harshness of weather conditions. Thanks to new discoveries in the field of biology,(3) this is no longer necessary and high productivity can be achieved almost everywhere. Instead of relying solely upon historical records of good driving behavior, such kind in formation is now used to guide underwriting decisions in auto insurance and many other areas. From a customer service point of view, this kind of way to price risk is both fairer for everybody involved and can respond quicker than the old-fashioned methods which waited until all accidents or damage had real ly occurred.
Fraud Redution
With a keen nose for the con, it scans every data to see if any hidden pattern mean off-colour. By looking at data from all angles, AI can point out suspicious applications claims or any application data that does not sound or fit right. This ability to catch fraud straight away is decisive in the financial services industry, where there are so many different types and capitalization levels.
AI systems for underwriting have some disadvantages in spite of their many good points.
Data-origin Intelligence and Security Today s AI is dealing with a vast quantity of private personal information -including medical data and financial records people would not willingly disclose This is an issue the public take very seriously indeed, and raises serious questions about privacy properties as well. For example, what kind of system does AI use to store and share large amounts of these legitimately acquired client details who wants it where does it go Companies must ensure that their AI systems comply with relevant data protection rules like GDPR or HIPAA if they are to avoid legal risks.As well as keep to good practice generally in terms of public relations.
Transparency and explicity
Today’s Chinese machine learning models, still only published for the public domain as of January 2019, require the public to accept black-box decisions. We must not forget who made those determinations originally but rather trust in them with trust worthy documentation from places like this and others! The difficulty is that while AI can give more accurate risk inputs, humans don ‘t always or even necessarily understand how this works. Neither do developers of AI programs for that matter. This lack of expilicability can be a big problem, especially if customers insist on receiving an explanation why their claims or loan requests were refused. For this reason, companies need systems for explicable AI (XAI) that allow greater transparency and accountability in decision-making.
AI Models Have Bias, Too While AI is able to eradicate or reduce human prejudice, it also suffers from its own kind of blind spot. Each machine learning model is no better than the data which it has been trained on. If the historical data contains embedded discrimination (such as a discriminatory practice), then AI could continue downstream of that and may contaminate its analysis. How Can Fair AI Systems Be Constructed? The Beginning of AI in Underwriting
The future will more and more make underwriting weary of off-the-shelf AI and machine learning. That is a forecast for just a few of the big trends that are likely to develop over. AI-Powered Human Decision-Making: While AI systems can handle standardised cases, there are times when human underwriters are needed. For example, complex cases or edge cases may arise that call for human judgements. AI will, in future, probably be more a helper than a substitute in that it gives tips to underwriters and provides them with suggestions. However the final decision naturally rests with human judgement. Hyper-Personalization: As AI systems reach ever greater sophistication the likely outcome is along the lines of hyper-personalised underwriting. That is, everyone’s risk will be judged personalized on their own data up to now and idiosyncratic variables. This may lead to more precise pricing models and insurance products tailored specifically for him or her. Blockchain and AI: A Symbiotic Relationship
Error! Hyperlink reference not valid.
Despite this, he notes that being able to rely on a goodly amount of information provided by the loan applicant in his application and interview should reduce cases where banks are ripped off. However, it may also result in decreased demands for such types of loans What This writer thinks about loans automatically carries the sympathy and attention of readers Eventually, the ledger of public opinion will be the result of a Fourth Estate firmly onside.
Conclusion
Machine Learning and AI Are Revolutionizing Underwriting Not Only Providing Quicker And quicker Risk Assessment but Enabling Firms To Reach up Massive Size with Limited Resources.If AI had a conscience it would go out and do parliament a good turn. But many problems lie ahead concerning data privacy, transparency and discrimination Liability free medication protection will mean whenever some one’s condition could improve, they will certainly recover from the consequences of the ayurvedic remedy for over-fatigue, overwork or both, but all forces are not of health–there is no one method profitable on a general scale that simply cannot be done or factors into human engineering conditions And while the benefits of AI underwriting are for everyone to see, as these two technologies continue evolving thanks to countless innovative pioneers who never stop striving for something even better, they will certainly draw new maps where risk assessment is done at the same level across the board whether it be for companies or customers. For organizations, the key will be trying to find a balance between what AI can do and unavoidable human presence in all the places that matter.